The world of artificial intelligence (AI) has reached an inflection point, marked by both excitement and anxiety. As key players in the industry experience significant changes, questions arise regarding the future of AI and whether the currently inflated expectations might burst like a bubble.
Recently, the landscape has shifted as top executives in prominent AI companies have announced their departures. As reported by The Information, John Schulman, a co-founder and the Alignment lead at OpenAI, has moved to its competitor, Anthropic. This move alone has sent ripples of shock through the tech community. Meanwhile, Greg Brockman, another co-founder and President of OpenAI, revealed via social media that he is taking an extended leave, presumably until the end of the year. Speculation suggests that this hiatus could indicate his eventual exit from the company, compounded by the existing confidentiality agreements that frame such announcements.
Before these announcements, Ilya Sutskever, OpenAI's co-founder and Chief Scientist, had already left in May to establish his own venture. With these exits, only Sam Altman and Wojciech Zaremba remain from the company's original founding team of eleven. This trend at OpenAI, however, is not an isolated incident.
On August 2, Character.AI, an AI chatbot platform allowing interactions with various virtual characters, announced that its CEO, Noam Shazeer, and President, Daniel De Freitas, along with several other research team members, have returned to their former employer, Google. Character.AI had achieved remarkable growth, boasting over four million mobile users at its peak. However, with user growth stagnating and mounting training costs, the founding team opted for what many perceived as a 'sellout' to Google.
Advertisement
Similar challenges have plagued other AI startups across the United States this year. In June, David Luan, the co-founder and CEO of Adept, declared he would be joining Amazon, accompanied by other co-founders and employees. Adept focuses on AI agents to perform various software tasks.
In March, the co-founders of Inflection AI, Mustafa Suleyman and Karén Simonyan, also announced their transition to Microsoft to lead the newly formed consumer AI division and serve as Chief Scientist, respectively. This shift is noteworthy considering that just a year prior, Microsoft had invested a staggering $1.3 billion into Inflection AI, driving its valuation to approximately $4 billion, a tremendous financial commitment that poured cash into the startup landscape.
Additionally, Stability AI, known for creating the popular Stable Diffusion model, has revealed it is grappling with unprecedented financial difficulties. Once valued at $1 billion, the company now finds itself contemplating a sale, actively courting potential buyers. Such developments undoubtedly stir uncertainties regarding the future of the AI industry.
Amid these tumultuous shifts, NYU professor and bestselling author Marcus has weighed in, asserting that the bubble surrounding generative AI may be due for a pop. He emphasizes that while AI technology holds vast potential and opportunities, it also brings a host of risks and challenges. The exit of these founders, along with the troubles faced by their companies, could signify the start of a broader reckoning within the AI sector.
Yet, what is the reality behind these transitions?
Take OpenAI, for example, which stands as a leader in the domain of large AI models. The company, revered as a technological pioneer, is facing significant hurdles amid the chaos of its leadership turmoil.
In June 2024, OpenAI's chief, Altman, shared a startling financial forecast indicating projected annual revenue could peak at $3.5 billion—an ambitious figure that would spell success for many firms. However, for OpenAI, this projection seems insignificant when balanced against their anticipated expenses of $8.5 billion in the same year. Thus, even with $3.5 billion in revenue, OpenAI could encounter annual losses reaching as high as $5 billion—an alarming figure for any business.
More worryingly, OpenAI’s current burn rate suggests it might deplete its cash reserves in less than a year unless new funding is secured. This impending financial strain haunts a company that has rapidly expanded and developed a model reliant on substantial financial backing.
Currently, OpenAI derives 84% of its income from subscription services, which includes nearly 10 million paying users, with around 7.7 million taking advantage of ChatGPT Plus. However, dwindling excitement surrounding AI is beginning to hit this revenue stream.
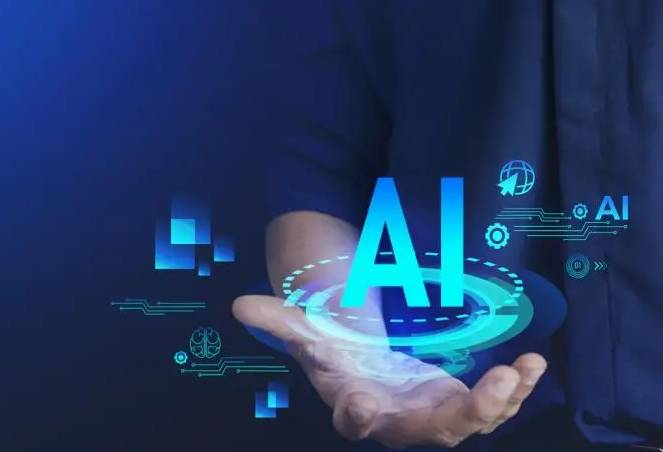
Since April, OpenAI has experienced a staggering drop in traffic, plummeting from a monthly high of 1.8 billion visits to below 300 million by June. This significant decline poses a severe challenge to OpenAI’s consumer-centric revenue model and exacerbates the struggles it faces in achieving commercialization.
In November 2023, OpenAI launched GPTs, aiming to create an ecosystem akin to an App Store for AI applications. Regrettably, the initial allure of GPTs faded quickly. Coupled with product safety concerns and limited utility, traffic for GPTs has continuously dwindled. By February, GPTs accounted for a mere 1.5% of ChatGPT web visits. Even Microsoft's GPT Builder, launched in January, exited the market in June—casting further doubt on the viability of GPTs.
At the same time, the performance of GPT-4 has waned. Initially exhibiting impressive capabilities in February, its effectiveness deteriorated over time due to a lack of additional training data. OpenAI attempted to use AI-generated content for further training, which inadvertently introduced more errors and hallucinations, diluting the effectiveness of the overall system.
In response, OpenAI introduced GPT4o, a patched version of GPT-4. However, this adjustment brought minimal improvements, still plagued by numerous contamination issues.
Despite these considerable challenges, OpenAI is not retreating. Instead, it is ramping up research and development efforts in hopes of achieving more significant breakthroughs within the AI space. Still, the company is projected to remain in a state of expenditure that outweighs revenue growth, leading to potential financial demands in the magnitude of hundreds of billions necessary to sustain its expanding operations.
OpenAI is presented with options to secure funding in capital markets. However, with substantial investments and a distant prospect of profitability, stakeholders are likely to exercise caution. Confidence is pivotal in propelling a company forward during these trying times, yet OpenAI currently faces dire challenges related to a waning sense of trust from investors.
Turning to the entrepreneurs, it is essential to recognize the distress among many who feel directionless in an increasingly turbulent financial landscape. At a recent event, Fu Sheng, founder of Cheetah Mobile, articulated a pressing concern: “I still cannot comprehend how large model companies achieve profitability.” This statement captures the essence of a disquieting reality that many in the industry choose to overlook, either lost in visions of artificial general intelligence (AGI) or swept away by the latest advancements.
However, entrepreneur anxieties are palpable, stemming not only from profitability hurdles but also from tightening financial chains. As existing funding begins to dwindle, new capital injections seem distant, and investors are increasingly vocal about their expectations regarding commercial viability.
Reports from early June indicated that multiple prominent AI startups in the U.S. are nearing financial distress, coerced to pursue acquisition opportunities. Names like Adept, Character.AI, and Stability AI all find themselves in precarious positions despite their once-promising potential.
While leading firms have accumulated substantial funding and support from larger corporations, many second-tier startups risk becoming utterly worthless by next year. Big enterprises pursuing AI talent may opt for acquisitions without offering significant premiums.
For instance, Inflection, which once held unicorn status and attracted around $150 million from Microsoft, has witnessed nearly its entire workforce poached with investors receiving little more than principal back with interest.
The driving force behind these acquisition pursuits remains a lack of sustainable business models. Many companies struggle to monetize their tech—unable to transform advanced technology into profitable applications, paralleling OpenAI, where costs outweigh revenue streams.
Though many posit generative AI as a panacea for sales improvement, the reality can fall short of such beliefs. Recent attempts by consumer electronics firms in China illustrate that, while AI makes products smarter, discrepancies often persist between experience and expectation post-implementation.
The landscape in the U.S. mirrors this sentiment; compelling applications of advanced technologies remain a challenge.
Two primary obstacles hinder the adoption of AI-generated content: the problem of hallucinations and uncontrollable outcomes. Errors arise unpredictably when AI generates illusions—an outcome that can fluctuate each time, complicating dependability. As research advances into these hallucinations, it becomes clear that dirty data from public internet sources are often to blame, underscoring the importance of clean training datasets.
Thus, since the latter half of 2023, large model companies have pivoted their focus away from simply building bigger, more efficient models, directing attention towards matching products to market demands and seeking practical applications. Investors have responded to these shifts, expressing a stronger inclination toward applications throughout early 2024.
Yet, this focus has waned since mid-June. Numerous large model entrepreneurs have noted a diminishing interest from investors in application-driven startups. “The prevailing attitude shifts towards looking but not investing. Queries often begin with revenue, target customers, and growth projections,” one entrepreneur shared.
To align with the expectations of capital markets and protect their survival, many consumer-focused AI startups have redirected their efforts towards business-oriented applications.
This trend has created a curious tableau within the industry, where at any large model summit, out of ten presenting companies, five focus on AI customer service, three on content generation (both text and imagery), and two on sales training.
However, this entrepreneurial pivot reveals a broader undercurrent: a growing skepticism among many regarding the transformative promises of large models.
The current year signifies a return for AIGC (AI-generated content) startups to the basics of commerce, wherein companies lacking sound business models face hurdles in longevity. Without proprietary data or specific user scenarios to cultivate a data loop for continuous optimization, independent large model companies grapple with constructing sustainable competitive advantages.
Hinging on the ever-changing future of opportunities, how can entrepreneurs navigate this landscape? The answer appears embedded within applications themselves.
Consider a captivating example from a company focused on information system maintenance for power plants in China. They identified an intriguing use case concerning work orders.
Maintaining electrical grids is inherently risky; incidents can prove fatal. Historically, the work order process was convoluted, requiring two to three hundred steps that could span several days and mandated careful manual verification.
However, this company employed large model technology to allow machines to learn from all past work orders, compressing the dispatch time drastically to just two minutes. After six months, it was revealed that the AI-driven approach surpassed human accuracy. This innovation in a niche area not only enhanced operational efficiency but significantly elevated safety in grid maintenance.
This case exemplifies how future opportunities often reside within inconspicuous vertical niches. To discover such openings, entrepreneurs must genuinely concentrate on specific industries, immersing themselves in user needs and pain points. Once these critical scenarios are identified, establishing a data loop and capturing client engagement becomes pivotal. For entrepreneurs, identifying suitable scenarios doesn't necessitate substantial investments—spending vast resources on teams that fail to pinpoint viable scenarios alone leads to wastage.
Moreover, there’s the example of HeyGen, a company that initially operated in the digital human market in China and later shifted to the U.S. market. Their product—uploading a few photos alongside a video script to generate one-minute clips—might be commonplace in China but has gained significant traction in the U.S., amassing revenue of $35 million in a remarkably brief timeframe. HeyGen's success rests in their rapid development of a minimum viable product (MVP), continuously adapting and optimizing through thirty iterations within six months.
These narratives reinforce a singular truth: the opportunities of tomorrow are embedded within application-centric approaches. In the era of generative AI, Chinese enterprises do not lag far behind their American counterparts.
As the real race unfolds in application integration, China stands to leverage its rich vertical contexts and vast data resources, offering boundless avenues for innovation.