In the world of finance and technology, few companies have made waves like Nvidia. On June 5th, the tech giant left the investment community abuzz when it saw its stock price soar to unprecedented heights. By the close of trading that day, Nvidia's stock had climbed by 5.16%, establishing a new historical record. Its market capitalization surged to a staggering $3.01 trillion, positioning it just behind Microsoft, which stands at $3.15 trillion, and ahead of Apple, a long-time powerhouse.
In a remarkable timeline, Nvidia achieved the milestone of moving from a $2 trillion to a $3 trillion valuation in merely three months. To illustrate the scale of this growth, Nvidia's stock has skyrocketed more than 147% year-to-date, following a breathtaking rise of 200% in the previous year. Such performance not only dazzles investors but also piques the curiosity of market analysts, who are eagerly optimistic about Nvidia's trajectory.
Mizuho Securities, reflecting this confidence, adjusted Nvidia's target price from $1,180 to an impressive $1,275, maintaining a "Buy" rating. Analyst Vijay Rakesh expressed high hopes for the company's future, emphasizing the potential of Nvidia's Hopper platform and the anticipated launch of the groundbreaking Blackwell B100 GPU within the coming months, which he believes will soon redefine the boundaries of artificial intelligence.
A few days prior, Nvidia's CEO, Jensen Huang, captivated audiences at the COMPUTEX event in Taipei, where he unveiled the 'Blackwell' chip. He passionately touted it as "the most complex and powerful chip ever created," designed to connect two die through NVLink switches and enable unparalleled computational power for generative AI applications with billions of parameters.
Advertisement
Huang didn't stop there. He introduced another innovation, the 'DGX Blackwell' system, capable of handling the computational needs of an entire AI company. With 72 Blackwell GPUs linked via NVSwitch, this machine epitomizes immense computational capability.
At the conclusion of his two-hour presentation, Huang showcased a striking iron frame, proclaiming it "the spine" that connects everything, housing up to 5,000 cables extending over two miles in length. He envisaged a monumental data center dubbed the "AI Factory," facilitated by interlinking multiple DGX Blackwells through this spine.
Despite Huang rehashing many points from Nvidia's March GTC conference, his fervor struck a chord with the financial markets, which remained eager for what lies ahead. As analysts ponder the extent of Nvidia's innovations, Huang's resolute dedication to progress has become an evident hallmark of his leadership.
What distinguishes Nvidia from other tech conglomerates like Apple? Huang has been clear in communicating that the trajectory towards ubiquitous artificial intelligence is only beginning, with both billion- and trillion-parameter models being just a foundation. The current developers must significantly amplify their investment and efforts to gain a competitive edge in this rapidly evolving field.
Most existing models leverage Google’s transformer architecture established in 2017, which, while beneficial, comes with substantial computational demands for training and deployment. A recent report from The New York Times revealed that OpenAI's ChatGPT requires approximately 30,000 Nvidia A100 GPUs and consumes over 500,000 kilowatt-hours daily to operate.
Even the hitherto celebrated "Mixture of Experts" (MoE) approach offers only marginal tweaks to this foundational architecture. The current race among various organizations still relies heavily on the brute force of computational resources to build and refine AI models.
Elon Musk has openly acknowledged that Tesla may spend up to $4 billion this year on Nvidia's processing units to bring its own AI models to fruition. Meanwhile, Huang is committed to pioneering solutions that lower the costs of utilizing transformer models, aligning Nvidia’s chip evolution with the market's increasing appetite for cost-effective computation.
There is no mistaking that fierce competition among tech giants drives demand for Nvidia's latest GPUs. Analysts suggest that unless there are revolutionary new frameworks, the future of AI model development will largely hinge on accumulating computational prowess. By 2025, demand for Nvidia’s GPUs could exceed a staggering one million units based on current A100 capabilities.
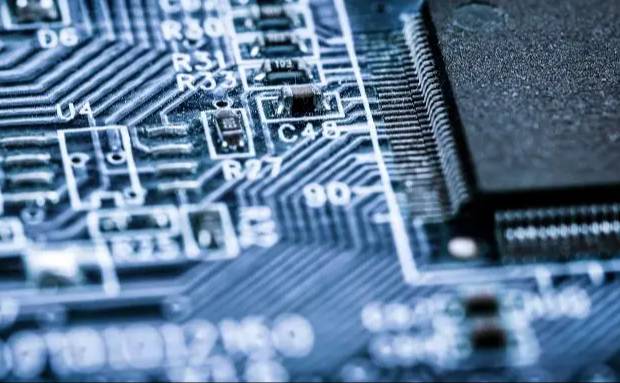
While competitors are racing to establish their own chip designs, Nvidia's dominance rooted in its CUDA platform gives it a competitive edge that others are keenly aware of. This dynamic creates a paradox where major internet companies must redirect resources to develop their own processing units while simultaneously investing heavily in Nvidia to remain competitive.
Adding a touch of irony, Huang excels as a marketer; each iteration of Nvidia's processing capabilities corresponds with a significant price increase. The A100 chip initially priced at around $3,500 has jumped to between $5,000 and $8,000, while the recently launched H100 retail starts at nearly $20,000 and projections for the GB200 chipset peg it above $40,000.
With demand pushing prices higher, Nvidia's stock has experienced significant recovery since earlier declines and is now tracking closely to Apple's market cap while narrowing the gap with Microsoft.
But can this growth be sustained? Huang is making substantial bets on the future.
Huang's recent speeches reflect a clear understanding of the AI landscape, with Nvidia’s market strategy predicated on his insights into the evolution of artificial intelligence and the specific sub-sector known as AIGC (AI-Generated Content). In his eyes, the big models currently dominating the news cycle, including ChatGPT, represent just the beginning of this innovation journey, with abundant opportunities available to those prepared to climb the ladder of advancement.
This optimism is evident, as Huang frequently emphasizes the potential for AIGC technologies while continually promoting various initiatives across social media channels.
As the market anticipates exponential growth in computational demands paralleling advancements in AI models, Nvidia’s prospects look increasingly promising.
Unlike Apple or Microsoft, whose focus remains on consumer sectors, Nvidia operates in the business-to-business domain, compelling it to present investors with expansive market prospects. Without this, Nvidia could find itself overly constrained compared to consumer-centric firms like Apple.
This is likely why Huang consistently champions the significance of leading internet companies developing robust models—yet this path is fraught with risks. Even the most minor shifts could lead to Nvidia's decline.
On one hand, even trendsetting companies like OpenAI and the various models from META and Google have yet to establish stable revenue streams from their offerings.
There are reports stating that OpenAI's monthly income covers less than one-third of its operating costs. Faced with challenges in defining a viable business model and rising hardware costs from Nvidia, management at these tech giants is beginning to question the validity of a model that relies heavily on resource inputs.
For these western internet giants, if their business models remain ambiguous, the long-term sustainability of high-resource investments is questionable. Currently, trailblazers in AIGC, including OpenAI, Microsoft, and Google, continue to grapple with establishing clear and consistent monetization strategies.
This uncertainty affects their willingness to commit resources in the long haul.
On the other hand, the focus of AI research in the west, particularly the U.S., might be misaligned. Historical trends dictate that demand should derive from applications, with innovation rooted in tangible needs.
However, much of the model development in the U.S. is focused more on theoretical understanding than practical user demand, leading to disjointed innovation.
This disconnect can be attributed, in part, to the hollowing out of industries due to virtualization, positioning the internet as capital’s only significant export from the U.S. The tech developers are consequently fixated on web-based applications over addressing real-world needs.
However, this trend is inherently flawed.
As questions regarding the cyclical nature of "which came first, the chicken or the egg?" arise, many in the U.S. find themselves banking on their self-developed models to eventually have reasoning abilities akin to humans, enabling them to assist factories, enterprises, and educational institutions.
Ignoring ethical considerations and technical hurdles along the way, the current AI models' propensity for errors raises concerns about their utility in everyday activities. If an AI like GPT makes over 10% of fabricated claims and mistakes, can it truly function effectively in a supportive role?
Moreover, while a graduate armed with knowledge is valuable, real-world experience is equally essential for effective application in professional settings. The insights drawn from operational and application data are crucial for successfully implementing AIGC initiatives targeting personalization.
It is ironic that gathering pertinent industry data in the U.S. is considerably more challenging than in China. The absence of a robust core statistical system and centralized government initiatives leads to fragmented industry data that is often confined to specific states.
The industry data required for effective AI model training and learning extends beyond operational metrics, necessitating comprehensive datasets from fields such as healthcare, including various diagnostic reports and imaging standardizations.
Legal discrepancies across states further complicate data acquisition, making it near impossible to derive cohesive national datasets.
Consequently, U.S.-based AI developers, including OpenAI, rely predominantly on publicly accessible internet data for model training purposes.
Under these conditions, even if the next generation of AIGC achieves human-like cognitive capabilities, developers will need to source authentic and holistic data for meaningful insights into their respective fields, lest they produce a form of knowledge that lacks operational efficacy.
Moreover, the specialized information required for AI deployment must encompass a broad spectrum to mitigate risks associated with inadequate data, especially in realms like healthcare, where unaddressed gaps in understanding can lead to disastrous consequences.
This illustrates the dilemma facing current U.S. model development efforts, threading the needle between innovation and application.
Under the surface of mounting successes lies a complex web of challenges. If the relationship between the "chicken and the egg" isn’t clarified, establishing a viable business model will remain elusive. In this context, the reliability of steady demand for Nvidia's GPUs from the major tech players is highly uncertain.
Adding to Huang’s difficulties is the erosion of Nvidia's market, particularly as they have gradually abandoned nearly half of its customer base in China. The geopolitical climate makes such a loss hard to swallow.
Chinese markets previously accounted for a quarter of Nvidia’s revenue, but due to political conditions, this has now plummeted to under 4%. To cover this shortfall, Nvidia must compensate with price increases for its new products.
The rapid advancements seen in China, with core models evolving at a pace surpassing that of their American counterparts, signal a shifting landscape. With innovative models such as Tongyi Qianwen's 110 billion parameters, Zhipu AI's open-source projects, and Wenxin's models carving out top positions internationally, the competition has intensified.
Furthermore, due to geopolitical developments in mid-2023, several major Chinese model developers are now stockpiling Nvidia's GPUs, resulting in excess computational capacity and sparking a price war within the burgeoning AI sector.
Taken together, these insights cast doubt on Nvidia’s long-term market viability amid such challenges.
As Cao Xueqin aptly noted in Chapter 13 of “Dream of the Red Chamber”: “We anticipate joyous news, reminiscent of fiery flames and flowing flowers.”
However, behind the moments of festivity lies the impending disintegration of the Jia family, a stark reminder of the impermanence of success.
In essence, what appears as rapid growth could easily morph into a perilous fall from grace.
It remains to be seen whether the market possesses the capacity to support Nvidia reliably.